On March 27th, 2020, just days after India went into a nation-wide lockdown to curb the spread of COVID-19, the Reserve Bank of India (RBI) announced a series of relief measures that included a moratorium of three months (March 1st – May 31st, 2020) on all term loans that lending institutions were encouraged to extend to their borrowers.[1] This was extended further for a period of three months (June 1st – August 31st, 2020) on May 23rd, 2020, because of the prolonged nature of the pandemic and the lockdowns it necessitated.[2] While we have already commented on the effectiveness of this six-month moratorium on financial service providers (FSPs) through our policy brief, in this study, we shift our focus towards borrowers and how they availed the moratorium. The objective of the exercise—through a case study—is to explore the extent to which different segments of borrowers availed the moratorium, and through that identify how much they may have been impacted by COVID-19 and lockdowns. We think that such an exercise can help FSPs in identifying the segments of their borrowers that need moratorium, restructuring, or other kinds of assistance during subsequent wave(s) of COVID-19 that are being anticipated, or other similar shocks.[3]
About the MFI, Data, and Methodology
We use the Customer Management System (CMS) data[4] from a non-banking finance company (NBFC) for the purpose of this case study, making use of the loan repayment information as well as the financial and demographic information of borrowers and their households.[5] The NBFC under consideration caters mainly to low-income segments from remote, rural areas who are otherwise outside the ambit of formal financial institutions. It has around five-lakh active borrowers in the states of Tamil Nadu, Chhattisgarh, Odisha, Uttarakhand, Karnataka and Jharkhand. We begin our analysis by identifying the various factors (state, income level, occupation, education level, etc.) that can be considered while segmenting the borrowers and check how each of the resulting segments availed the moratorium. As a next step, we carry out regression analysis to check which of these factors had an influence on the extent to which borrowers availed the moratorium, as well as the kind and degree of influence they had.
- Segmentation Analysis
The NBFC initially extended the moratorium across the board as it needed time to prepare to contact borrowers virtually to inform them about the moratorium, how it would affect interest, principal, tenure, etc., as lockdown restrictions ruled out physical outreach. So, we find almost all the borrowers to have availed the moratorium for at least a month (98.6%).[6] Gradually, as lockdown restrictions eased and more borrowers could be reached physically or virtually, they were encouraged to begin repaying if they could afford to, by opting out of the moratorium. Accordingly, we find that a majority of borrowers (60%) availed the moratorium partially (2-4 repayments), while a tenth of them hardly availed it (made 5-6 repayments), beginning repayments as soon as restrictions eased. But, nearly a third of the borrowers continued to avail the moratorium throughout, making at most one repayment (Figure 1).
Figure 1: Take-up of COVID-19 moratorium by all MFI borrowers (March to August, 2020)

Next, we look at state-wise variation in moratorium take-up (Figure 2). The share of borrowers availing the moratorium fully is higher in Odisha (58%) and Uttarakhand (48%), and much lower in Jharkhand (15%) and Karnataka (18%). On the other hand, more than a fifth of borrowers from Chhattisgarh (21%) hardly availed the moratorium.
Figure 2: Take-up of COVID-19 moratorium by borrowers from different states
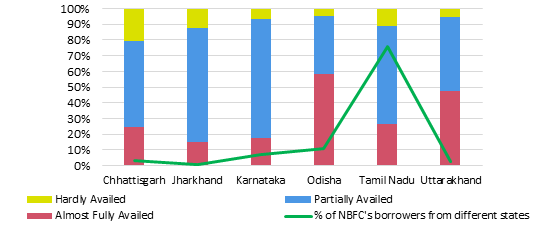
We find marginal differences in how borrowers involved in different occupations availed the moratorium (Figure 3). A higher share of borrowers involved in business[7], informal work[8], and those unemployed[9] availed the moratorium fully, while a lower share of those involved in agriculture[10], livestock[11], labour, salaried jobs, and dependent on rent/remittance availed it. Even when it comes to borrowers belonging to different income and education levels, there are only marginal differences in take-up (Figures 4 and 5). The share of borrowers fully availing moratorium reduces—and the share of borrowers hardly availing the moratorium increases—as we move to higher income and education levels.
Figure 3: Take-up of COVID-19 moratorium by borrowers involved in different occupations

Figure 4: Take-up of COVID-19 moratorium by borrowers from different income quintiles[12]

Figure 5: Take-up of COVID-19 moratorium by borrowers with different educational levels
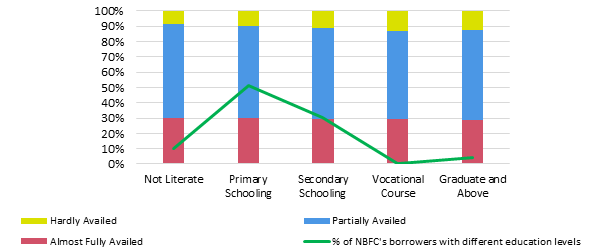
2. Regression Analysis
We follow up with a regression analysis to check whether and how each of the factors we consider for segmentation influenced the number of repayments made by the borrowers, i.e., the extent to which they availed the moratorium. Table 1 gives a summary of model specifications, dependent and independent variables, and their results.[13]
Both the models give similar results in terms of the impact the independent variables had on the number of repayments made by the borrowers during the COVID-19 moratorium, i.e., the extent to which they availed the moratorium. The likelihood of borrowers having made more repayments during the moratorium increases with higher income quintile, ownership of a greater number of vehicles and formal savings/investment instruments, and the education level of the borrowers. On the other hand, the likelihood of borrowers having made more repayments decreases with increase in the size of borrowers’ household, the number of active loans they held, and the number of physical assets they had to maintain.
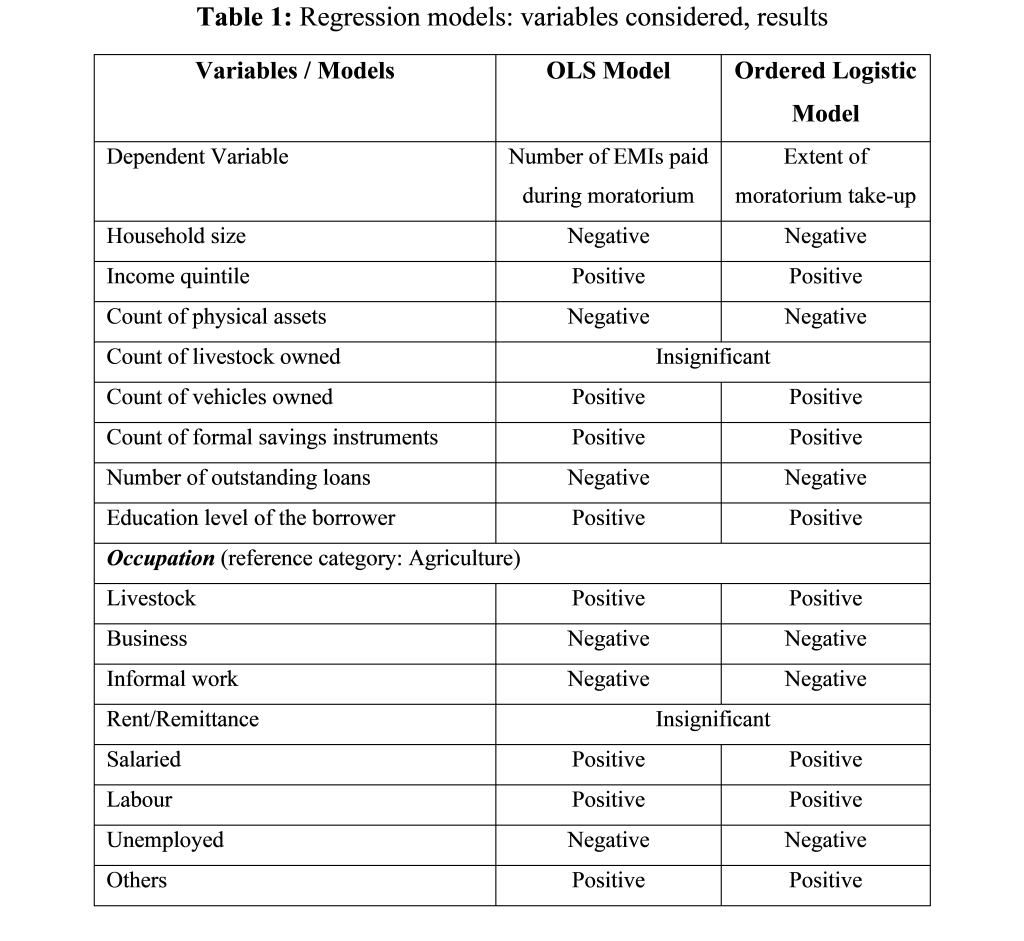
In terms of occupation, the repayment behaviour of those involved in agriculture (Figure 3) is very similar to the overall repayment behaviour (Figure 1), and of those dependent on rent/remittance is also not significantly different. Keeping it as a reference, those involved in livestock, labour, and salaried jobs have a higher likelihood of having made more repayments, while those involved in business, informal work, and those unemployed have a higher likelihood of having made fewer repayments.
All of these findings are intuitive and/or in line with the literature and anecdotal evidence on the impact of COVID-19 on low-income households and livelihoods. Ownership of immovable assets has a negative relationship with number of repayments made, whereas ownership of moveable assets like formal savings instruments has a positive relationship, indicating that the latter may have helped the borrowers to an extent in coping with the shock by giving them easier access to liquidity. The number of active loans held also has a negative relationship with number of repayments and this is in line with anecdotes from executives of lending institutions, of many borrowers preferring to repay informal loans first, since they had the luxury of moratorium for formal loans. Another factor that has a negative effect on repayment is the size of the household, and this is understandable since larger households tend to have more dependents and necessitate a higher consumption expenditure.[14] Those involved in agriculture and livestock seem to have availed the moratorium to a lesser extent than those involved in business. While agricultural and livestock activities were still permitted during the lockdown and agriculture was one of the only sectors registering positive growth during this period of overall negative growth,[15] most of the business activities faced harsher restrictions, including partial or full closure. Those having relatively safer, formal, salaried work seem to have made more repayments than those involved in informal work.
Conclusion
The first wave of COVID-19 pandemic was a long and unprecedented shock to Indians, especially to the low-income households. The six-month debt moratorium was announced by the RBI as an immediate relief measure to help them smooth consumption, at a time when their lives and livelihoods were under distress due to the pandemic and lockdowns respectively. The evidence from this case study of almost five lakh microcredit borrowers from an NBFC shows that almost all of them availed the debt moratorium at least partially.
There is a noticeable difference in take-up of moratorium between states, with a substantially higher share of borrowers from Odisha and Uttarakhand availing it fully, while the share of borrowers who hardly availed the moratorium is higher in Chhattisgarh. The high moratorium take-up in Odisha and Uttarakhand may also have been because of other natural calamities that also affected the two states during the same period, or because of their dependence on migrant remittances and tourism, which were both affected during this period.[16][17] But there are only marginal differences in take-up between borrowers involved in different occupations and belonging to different income and educational levels. Nevertheless, the regression analysis shows that the degree to which borrowers availed the moratorium significantly depended on all these factors. While the likelihood of borrowers having made more repayments increases with increase in income and education levels, ownership of moveable assets, and formal savings instruments, it decreases with increase in size of the household, number of outstanding loans held, and number of immoveable, physical assets owned. Those involved in agriculture, livestock, labour, salaried work, and those dependent on rent/remittance have a higher likelihood of having made more repayments than those involved in business, informal work, and those unemployed.
These results show that the less financially well-off borrowers and those who found themselves in greater distress during the period availed the moratorium to a greater extent. There is merit in FSPs carrying out similar analysis to assess the heterogenous impact such shocks have on different segments of their borrowers, so that it can help them in identifying segments that may need more assistance, in the form of moratorium, restructuring, etc.
Annexure: Regression Analysis
- OLS Regression Model:
First, we fit a simple OLS regression model on the number of repayments made by the borrowers during the moratorium period against a set of independent variables. The formula for the regression model is as specified below and the results are shown in Table 2.



The low R-squared value of 0.04 means that the effect of all our independent variables on the dependent variable is only marginal, although statistically significant. Another reason for the low value is because of high variability in the large, noisy dataset. A constant score of 2.63 means that the model predicts that a borrower made around three payments during the moratorium period if all independent variables for her are kept at zero. The co-efficient value of a predictor variable explains how a one unit increase in it changes the predicted number of repayments made by the borrower, provided all other independent variables are held constant. For example, the co-efficient value of -0.16 for household size means that an increase in size of a borrower’s household by one unit reduces her predicted number of payments by 0.16, provided all other factors are held constant.
Except the number of livestock owned, all other independent variables have a significant effect on the number of repayments. In addition to the income quintile to which the borrower’s household belongs and the education level of the borrower, the number of formal savings instruments and vehicles owned by the household have a positive relationship with the number of repayments made. On the other hand, the size of the borrower’s household, the number of outstanding loans held, and the number of physical assets owned have a negative relationship. In terms of occupation, borrowers involved in all occupations except those dependent on rent/remittance show a significant difference from those involved in agriculture (reference category). The model predicts those involved in livestock activities, labour, and those having salaried jobs to have made more repayments than those involved in agriculture, while those involved in informal work, business, and those who were unemployed are predicted to have made lesser repayments.
Robustness Check: We check the model for multicollinearity between any of the independent variables by inspecting their variance inflation factors (VIF). A VIF value lesser than 10 and tolerance greater than 0.1 for a variable means that it could be a linear combination of other independent variable(s) considered in the model. All the independent variables in our model have a VIF < 10 and tolerance > 0.1, thus ruling out any multicollinearity (Table 3).

2.Ordered Logistic Regression Model
Alternatively, we fit an ordered logistic regression model on the degree to which borrowers availed the moratorium (fully availed, partially availed, hardly availed). The formula is as specified below and the results of which can be seen in Table 4.

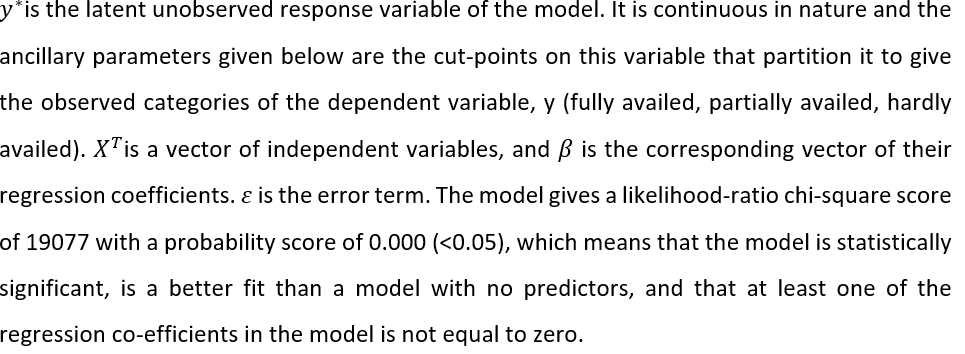
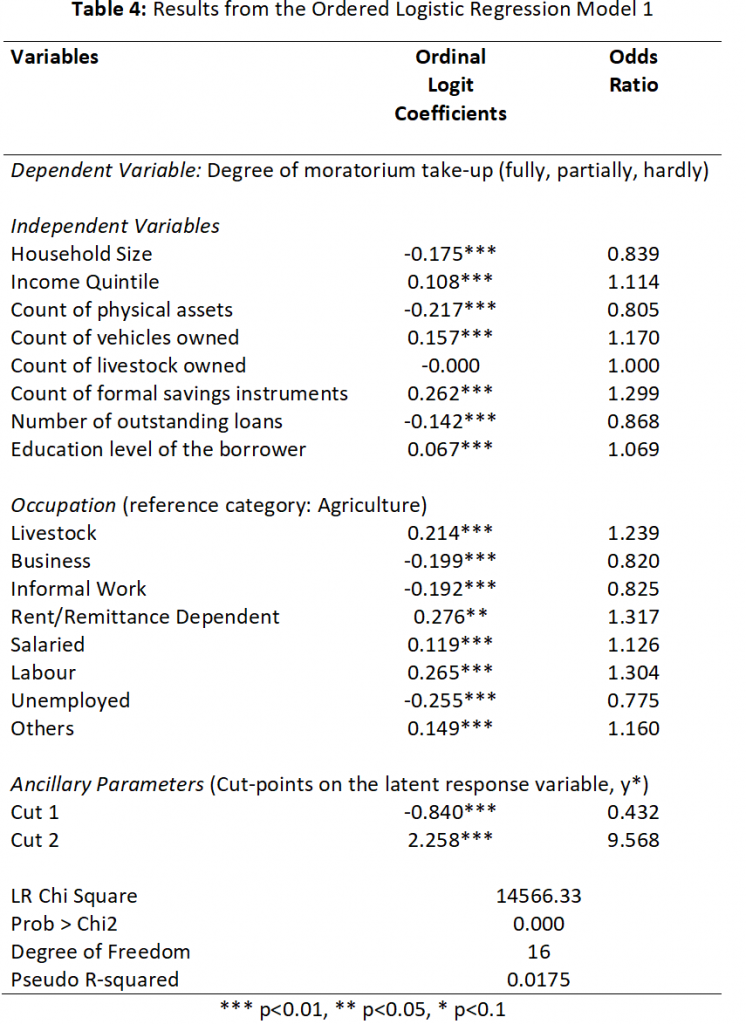
The co-efficients associated with each independent variable indicate the change in log odds of a borrower making more/less repayments given a one unit increase in the corresponding independent variable, provided all other independent variables are held constant. Similarly, the odds ratio gives the change in odds of a borrower making more/less repayments given a one unit increase in the corresponding independent variable.[18] For example, the co-efficient value of -0.17 for household size means that a one unit increase in a borrower’s household size reduces the latent continuous response variable score in log odds scale by -0.17, if all other values are held constant. The odds ratio of 0.84 associated with household size means that for each unit increase in household size, the odds of a borrower making more repayments reduces by a factor of 0.84.
Similar to the results from OLS model, the likelihood of borrowers making more repayments increases as we move to higher income quintiles, education levels, ownership of more formal savings instruments and vehicles, while it decreases with increase in size of the household, ownership of immovable physical assets, and number of outstanding loans. Even in terms of occupation, the results are similar to those from the OLS regression model.
The ancillary parameters indicate the cut-points in the latent response variable (y*) to map its continuous scores to the corresponding ordinal categories of the dependent variable (y). For example, if the latent response variable for a particular borrower is below -0.84, the model maps it to the ordinal category of fully availed and predicts that the borrower fully availed the moratorium.
Robustness Check: We check the model for proportional odds assumption that is central to ordinal logistic regression.[19] This is done by first regressing the model without imposing the proportional odds assumption on any of the independent variables, and then by regressing the model again by imposing the assumption on all variables. The significant p-value (Table 5) on the likelihood ratio test implies that the model satisfies the proportional odds assumption.

Appendix
Plot 1: Take-up of COVID-19 Debt Moratorium by Loan Type
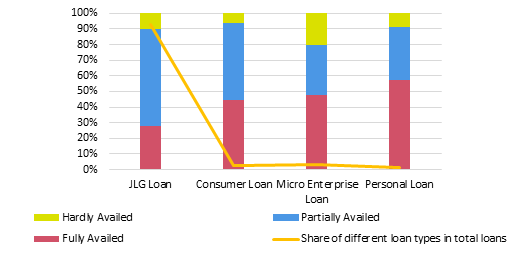
[1] RBI Notification on Debt Moratorium dated March 27, 2020
[2] RBI Notification on Extension of Debt Moratorium dated May 23, 2020
[3] The impact of different shocks will be heterogeneous for different segments of borrowers, but carrying out such analysis regularly gives the FSP an indication of the extent of distress these segments face during different kinds of shocks.
[4] All active loans disbursed before March 2020 were considered for the analysis
[5] Loan related information of customers is updated regularly in the CMS, while their financial and demographic information is collected at the time of their enrollment into the NBFC and during each round of disbursement
[6] 1.3% of borrowers made all the repayments during the moratorium period. Some of them may have made use of digital repayment option, as during the month of April at least, physical collection of loan EMIs by wealth managers was halted because of the lockdown
[7] Business: Those who have reported their occupation as business, shop owner, small industry, and tailoring
[8] Informal work: Those who have reported their occupation as driver, performing arts, and migrant labour
[9] Unemployed: Those who have reported their occupation as house-wife, student, and unemployed
[10] Agriculture: Those who have reported their occupation as agriculture and agri-trading
[11] Livestock: Those who have reported their occupation as dairy, fishing, and goat-rearing
[12] Income quintiles are constructed based on annual household income self-reported by borrowers
[13] A detailed summary of regression tables, specifications, results, interpretation, and robustness checks are presented in Annexure 1
[14] Many migrants also returned back during the same period, putting additional burden on households
[15] How agriculture grew despite COVID crisis (India Spend)
[16] Super cyclone Amphan leaves 45 lakh affected in Odisha
[17] 2020 pre-monsoon rains hit farmers hard: Uttarakhand
[18] The odds ratio value of an independent variable is the exponential value of its co-efficient in log odds scale
[19] The proportional odds assumption means that for each term included in the model, the slope estimate between each pair of outcomes across two response levels are assumed to be the same regardless of the partition considered (Understanding the proportionality odds assumption)
Cite this item
APA
Ponnathpur, R. S. (2021). How Microcredit Borrowers Availed the COVID-19 Debt Moratorium: A Case Study. Retrieved from Dvara Research.
MLA
Ponnathpur, Rakshith S. “How Microcredit Borrowers Availed the COVID-19 Debt Moratorium: A Case Study.” 2021. Dvara Research.
Chicago
Ponnathpur, Rakshith S. “How Microcredit Borrowers Availed the COVID-19 Debt Moratorium: A Case Study.” 2021. Dvara Research.
2 Responses
Very interesting analysis Rakshith. What can we say about the efficiency (from a client welfare perspective) of moratoriums with this opt-out feature? Does it seem to be benefitting those with the highest need? Would be also helpful to track loan payment data for these customer groups post-moratorium. Are those who availed the moratorium also more likely to get a restructure?
Thank you, Bindu. We do see from the analysis that those from lower income quintiles, those owning fewer savings instruments, those involved in highly affected occupations during the COVID-19 first wave and related lockdowns, availed the moratorium to a greater extent. So, it does look like the opt-out moratorium feature was helpful for those in greater need and they availed the moratorium to a greater extent than others. Your suggestion about tracking loan repayment behaviour post moratorium and likelihood of being offered restructuring is very helpful. We are yet to do a follow-up study on this, but we shall look into it in the coming days.